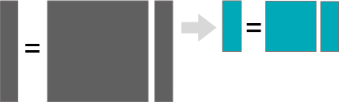
Surrogate modeling & model reduction
Cross-
Cutting
Research Area
Harnessing the data through the lens of physics-based modeling
Scientific Machine Learning brings together the complementary perspectives of computational science and computer science to craft a new generation of machine learning methods for complex applications across science and engineering. In these applications, dynamics are complex and multiscale, data are sparse and expensive to acquire, decisions have high consequence, and uncertainty quantification is essential. The greatest challenges facing society — clean energy, climate change, sustainable urban infrastructure, access to clean water, personalized medicine and more — by their very nature require predictions that go well beyond the available data. Scientific machine learning achieves this by incorporating the predictive power, interpretability and domain knowledge of physics-based models.
Research is multifaceted, ranging from foundational advances in theory, methods and algorithms, to real-world impact in societal grand challenge problems.
Surrogate modeling & model reduction
Bayesian inverse problems
Physics-informed deep learning
Data assimilation
Interpretable machine learning
Reinforcement learning
Digital twins
Optimal experimental design
The Oden Institute and The Alan Turing Institute have a memorandum of understanding to collaborate in the areas of artificial intelligence for science and engineering, computational science and engineering, scientific machine learning, and data-centric engineering. Established in 2015, The Alan Turing Institute is a high-profile, vibrant and multidisciplinary national institute, bringing together 13 leading universities from England and Scotland, making it the UK’s national institute for data science and artificial intelligence.
The Oden Institute's Center for Scientific Machine Learning has strong collaborations with Department of Energy programs, including the AEOLUS Multifaceted Mathematics Integrated Capability Center, the ARPA-E DIFFERENTIATE program for design intelligence, and the Artificial Intelligence and Decision Support for Complex Systems program. Our faculty also played a key role in the ASCR visioning report on Basic Research Needs for Scientific Machine Learning
.To learn more about projects and people in Scientific Machine Learning, explore the centers and groups with research activities in this cross-cutting research area.
Center for Scientific Machine Learning
Optimization, Inversion, Machine Learning, and Uncertainty for Complex Systems
Computational Research in Ice and Ocean Systems Group
Probabilistic and High Order Inference, Computation, Estimation, and Simulation
News
July 7, 2025
A research team led by UT Austin has achieved a 90,000x speedup in simulating next-generation rotating detonation rocket engines, dramatically reducing computation time. This breakthrough enables real-time engine design and optimization.
News
June 23, 2025
Willcox is the first woman to receive the Grand Prize, which is awarded by the Japan Society for Computational Engineering and Science.
Profile
May 15, 2025
With a passion for mathematics and her sights on the stars, CSEM graduate student Nina De La Torre is inspired to make research accessible to all audiences.
News
April 21, 2025
The award recognizes Oden Institute core faculty who have demonstrated a sustained record of distinguished research in computational engineering and sciences.
News
March 20, 2025
Students from Del Valle High School were hosted by the Oden Institute's Center for Autonomy to explore technological innovations at the frontiers of academia and industry.
Profile
March 18, 2025
Read about CSEM graduate student Graham Pash, his research at the Oden Institute, and motivating the next generation of mathematicians.
News
March 11, 2025
From flying paper planes to building robot hands, explore how the Oden Institute's hands-on activities at STEM Girl Day at UT introduced thousands of young learners to the world of computational science.
News
March 4, 2025
Omar Ghattas was awarded the Ivo and Renata Babuška Prize at the 2025 SIAM Conference on Computational Science and Engineering.
Profile
Feb. 21, 2025
Joe Kileel, Principal Faculty at the Oden Institute for Computational Engineering and Sciences, and mathematics professor was awarded a Sloan Fellowship in February 2025.
News
Feb. 12, 2025
Scientists and supercomputers generate fast storm surge forecasts in 2024 which are used to help protect lives and property.
News
Oct. 16, 2024
Three Oden Institute faculty members gave presentations at TACCSTER, the Symposium for Texas Researchers, held at the Texas Advanced Computing Center (TACC).
News
Oct. 14, 2024
The second annual Scientific Machine Learning Workshop served as an incubator for new perspectives and recent developments within the field, while connecting early career researchers with established experts.
News
Sept. 18, 2024
The new NSF-Simons AI Institute for Cosmic Origins (CosmicAI), led by Stella Offner, Oden Institute core faculty, will develop AI approaches to investigate the origins of the universe including the formation of galaxies, stars, planets and even the building blocks for life.
CosmicAI will be housed at the Oden Institute at UT Austin.
Profile
July 8, 2024
Get to know Gabrielle Costello, and learn about her journey from high school English teacher to research administrator at the Oden Institute.
News
May 16, 2024
In his talk, Quarteroni emphasized the symbiotic relationship between AI algorithms and physics-inspired numerical models.
News
May 14, 2024
The award recognizes Oden Institute core faculty who have demonstrated a sustained record of distinguished research in computational engineering and sciences
News
April 20, 2024
Del Valle High School students visited the Oden Institute's Center for Autonomy and experienced hands-on tours led by graduate students.
Feature
April 8, 2024
Read about the Center for Computational Oncology's work with the MD Anderson Cancer Center to design clinical trials for patients who are not average.
News
Feb. 23, 2024
Oden Institute Director Karen Willcox discusses the potential for digital twin technology during an interview for NPR's TED Talk Radio Hour.
News
Feb. 22, 2024
Published in Computer Methods in Applied Mechanics and Engineering, new research from the Oden Institute shows how digital twins can be applied to enhance the maintenance and safety of civil structures.
News
Dec. 31, 2023
The Charles Chui Award, accompanied by a cash prize and a certificate, holds significant prestige in the harmonic analysis community.
News
Dec. 15, 2023
Across multiple domains of science, engineering, and medicine, excitement is growing about the potential of digital twins to transform scientific research, industrial practices, and many aspects of daily life.
This report identifies the foundational research and resources needed to support the development of digital twin technologies.
News
Dec. 1, 2023
Published in the journal Frontiers in Artificial Intelligence, Oden Institute researchers show how methods developed for digital twins in aerospace engineering can be used for high-grade glioma patients undergoing radiotherapy.
Media Coverage
Nov. 15, 2023
By extracting ideas from different fields and applying them to broad problems, Rachel Ward has built unexpected connections across a huge swath of applied mathematics.
News
Oct. 23, 2023
Stella Offner, an Associate Professor of Astronomy at UT Austin, has transitioned from an affiliated to core faculty member within the Center for Scientific Machine Learning at the Oden Institute.
News
Sept. 7, 2023
The talk, originally delivered at the TEDxUTAustin Conference in March 2022, sheds light on how digital twins have revolutionized decision-making processes.
News
Aug. 2, 2023
The U.S. National Congress on Computational Mechanics awarded Karen Willcox with a distinguished medal and is named a USACM Fellow.
News
June 23, 2023
Tan Bui-Thanh recieved multiple grants for new research which aims to fix machine learning’s struggle with uncertainty.
Feature
June 5, 2023
A glimpse into visiting researcher Tammy Kolda's experience at the Oden Institute.
News
May 8, 2023
CSEM Ph.D student Shane McQuarrie was awarded the ninth BGCE Student Paper Prize at the 2023 SIAM CSE Conference.
News
April 19, 2023
Scientific Machine Learning (SciML) two-day workshop was a success, with nearly 100 in attendence.
News
Feb. 27, 2023
Researchers from UT Austin have been awarded a prestigious grant from the UK’s Wellcome Trust to understand how flood and storm surge modeling could help inform infectious disease spread in Texas and beyond.
News
Feb. 9, 2023
The new simulation took only 9.4 milliseconds to run on a laptop computer, one billion times faster than a traditional model.
News
Sept. 26, 2022
Feature
Sept. 12, 2022
Media Coverage
Sept. 1, 2022
News
Aug. 18, 2022
News
Aug. 8, 2022
News
June 16, 2022
Feature
May 18, 2022
News
May 18, 2022
Profile
May 17, 2022
Feature
March 21, 2022
News
Feb. 11, 2022
News
Sept. 22, 2021
News
Sept. 8, 2021
Feature
Aug. 31, 2021
Media Coverage
June 8, 2021
News
March 24, 2021
Media Coverage
Feb. 4, 2021
Media Coverage
Feb. 1, 2021
News
Jan. 21, 2021